Initial situation, Problem definition:
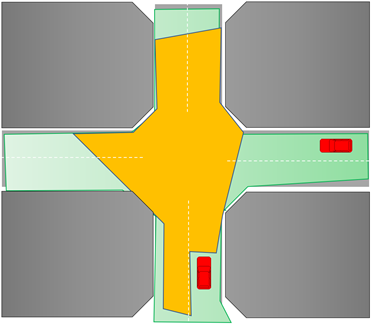
Future vehicles (autonomous or human piloted) cooperate via wireless networking to improve safety and reliability of road traffic. Each vehicle establishes a local view by means of sensors such as lidar, RADAR and cameras to detect other road users (pedestrians, bicycles, or other motor vehicles) as well as road obstructions. All mentioned sensors require a line-of-sight which is often blocked by buildings or vehicles and thus important information is missing in the vehicles local view. Radio waves can propagate around corners or other obstacles allowing to complete the vehicles local view by obtaining sensor data, object positions and traffic information from other road users. The reliable communication range for this data exchange varies largely depending on the local environment of the vehicle and is currently unknown to the vehicles local (autonomous) control or safety algorithms.
Goals and level of innovation:
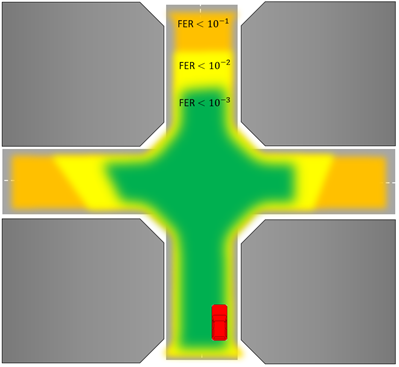
The project RELEVANCE aims to solve this uncertainty by: (i) Enabling a vehicle to predict the reliable communication region in real-time, i.e. the geographical region within which it can reliably exchange traffic and sensor information with other road users. (ii) Utilizing reliable communication region prediction for V&V (verification and validation) during the development of ADAS (advanced driver assistant systems) and AD (automated driving). Fast coverage prediction enables the generation and prioritization of the most relevant testing scenarios for V&V. Identified boundaries of coverage allow to efficiently steer tests towards edge cases, which are otherwise hard to find but are highly relevant for safety validation.
The central innovation of the project RELEVANCE is to investigated methods that enable the utilization of geometrical database information (streets, buildings, traffic signs and other obstacles) as well as vehicle sensor data to train a deep neural network for the prediction of the reliable communication region of a vehicle in real time.
Expected results and findings:
We will:
- investigate the real-time prediction of the reliable wireless communication region of vehicles. Using available processed sensors information from the vehicles’ sensors and a novel dynamic geometry-based stochastic channel model as the input to trained and validated deep neural network predicting the reliable communication range in terms of a FER (frame error rate) estimation.
- provide an open dataset on wireless channel measurements enriched with sensor data from a vehicle for coining a scientific challenge on the prediction of the wireless reliable communication region.
- develop HOPE (high-performance open-source computing reference framework) which allows to investigate supervised deep learning methods for reliable communication region prediction. The framework will consist of modules for channel modelling, FER estimation, sensor data provision (i.e., LiDAR or RADAR data), deep learning models and a simulation which enables vehicle-in-the-loop testing.